İşitme Cihazları ve İşitsel İmplantlarda Yapay Zeka Uygulamaları: Kısa Özet
- engindani5
- 7 Nis 2022
- 12 dakikada okunur
Güncelleme tarihi: 27 Nis 2022
APPLICATIONS OF ARTIFICIAL INTELLIGENCE IN HEARING AIDS AND AUDITORY IMPLANTS: A SHORT REVIEW
Abishek Umashankar, Anusha M.N., Pachaiappan C. Journal of Hearing Science · 2021 Vol. 11 · No. 3
Abstract
Artificial intelligence (AI) has been broadly used for a long time, but in terms of hearing aids it only came into the limelight in 2004. Although at that time AI was yet to be incorporated into a hearing aid, with improvement in technology, slowly AI began to be intro- duced. AI included trainable hearing aids, own voice processing, brain-controlled hearing aids, strategies to improve speech perception in noise, and wind noise management in both hearing aids and cochlear implants. This short review discusses these AI technologies and their utility for the user.
Introduction
Artificial intelligence (AI), often synonymous with machine learning, refers to computer expertise in simulating human intelligence as applied to problem-solving, logical reason- ing, and tackling complex problems [1]. In other terms, it is the intelligence exhibited by machines, compared with the natural intelligence of humans and animals [2,3]. It is a branch of computer science that involves developing computer programs to devise applications ranging from run- ning shoes, medical imaging, and robotic vacuum cleaners to navigation systems, and so on. These present a picture of ‘Science Fiction’ scenarios where robots play a signifi- cant role [3]. AI can accomplish tasks that demand human intelligence, for example, recognizing speech, seeing, trans- lating, and making decisions. AI is a broad combination of deep learning and machine learning which includes algo- rithms for self training, feature extraction, and prediction of future outcomes. Nevertheless, all AIs are not equally cre- ated. Among various levels, the most common is symbolic AI, where a human task is done by machine learning [4].
In the hearing aid and cochlear implant industry, AI tech- nology has become the focus of current research and a future trend in the industry. Hearing devices have proven they are successful solutions to hearing loss, and the use of AI to make these devices even better is extraordinary [5]. In meeting an individual’s needs, AI can improve the qual- ity of perception and enhance ease of use. AI is a techno- logical breakthrough that has improved hearing capabili- ties and advanced users’ lifestyles [6]. Current AI features are trainable hearing aids and own voice processing which enables automatic programming of a hearing aid based on the present environment. Future technologies include brain controlled hearing aids with the help of AI algorithms like DNN (deep neural network) and CNN (convoluted neu- ral network) [7]. Details of AI or machine learning algo- rithms in hearing aids and implants are discussed below.
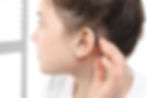
Material and methods
Articles published from various peer reviewed journals were searched in different databases: Medline/Pubmed Central, J-GATE, Google Scholar, and a manual Google search. Advanced search based on Boolean operators and keywords were used. White papers from various compa- nies were also considered. The latest technology from dif- ferent companies were searched on company websites and were included. The following MESH terms were used in the databases above: ((((((((artificial intelligence) OR (AI)) OR (deep learning)) OR (machine learning)) AND (hear- ing aids)) OR (cochlear implants)) OR (CI)) OR (hearing devices)) OR (auditory implants). Inclusion criteria were not kept strict as peer reviewed publications in this area are limited. Inclusion criteria were articles related to AI in hearing aids and cochlear implants, randomized control trials, clinical trials, case reports, and unpublished white papers. Articles related to AI algorithms in hearing devices were also considered. Articles on recent advances in hearing technology but not on AI were excluded. The data extrac- tion was done by two independent reviewers.
Results and discussion
A significant utility of AI technology is to enable a hearing impaired individual to perceive speech with better quality, especially in different environmental settings. Even though noise reduction algorithms already exist, an AI algorithm can automatically change its program settings based on the environment. It is said that AI technology can overcome the cocktail party effect in hearing-impaired individuals. AI technology also makes use of a hearing device easy. It gives form to the auditory intention of the user enabling them to achieve what they want [8].
Hearing aids with AI algorithms ‘learn’ the user’s hearing preferences, and via a set of observations and questions, it decides how one wishes to hear [9]. It uses complex pro- cessing to achieve a high-definition, natural sound which ensures that all the subtleties are heard. It reduces the need for manual adjustments by the wearer as it tailors and ori- ents the gain naturally. This has paved the way for many innovations such as Signia’s application of AI to ‘Own Voice Processing’ (OVP) [10]. This OVP features natural sound- ing of the wearer’s voice; it ‘learns’ to recognize the voice and processes it separately while external sounds are undis- turbed. This is a solution for those who cite the sound of their own voice as a drawback to using a hearing aid, and it only takes a few seconds [11].
There are many applications of machine learning, such as brain-controlled hearing aids that track the listen- er’s brainwaves and, based on a parallel with the source of sound in the environment, decide which speaker the listener is attending to. The device will then amplify the attended speaker relative to the others to facilitate hearing that speaker in a crowd. This is called auditory attention decoding (AAD) which automatically extracts the speaker from a mixed audio source. However, speaker-indepen- dent separation of speech is very challenging, and prog- ress towards a solution is difficult. Frameworks have been proposed for better performance, and one hopes it is just around the corner. Therefore for better speech detection, neural network models of the auditory cortex and audi- tory attention is the next focus. A critical component of such a system is a real-time, low-latency speech-separa- tion algorithm based on deep neural network models. These models approximate the computation performed by biological neurons, and have proven to be extremely effective in many machine learning tasks. The ongoing research in this area focusing on advancing our under- standing of auditory attention and its neural markers in the human auditory cortex. The aim is to remove the tech- nological barriers to AAD for improving speech intelli- gibility and reducing the listening effort in people with hearing loss [12].
Throwing light on another successful use of AI, machine learning has enabled hearing aids to detect speech and carry out language translation, track physical and men- tal health, and sense falls. Using recorded data, predic- tions can be made, making it possible to detect speech and provide an amplified translation to the ear in real time. Motion sensors can detect falls and then convey alerts and GPS locations to people such as caretakers or family members. These hearing aids are even intelligent enough to monitor spatial locations and log them accord- ingly. Later, it can use the GPS to determine when the user goes back to the same places and can send a notification if they want to adjust or automatically change preferred settings. Automatic preference adjustment in both quiet and noisy situations gives better speech perception based on the spatial sounds present [6a].
Machine learning or integral algorithms predict outcomes with learning experiences resulting from an individual or human input [13]. The best example is the Widex AI based upon the “SoundSense Learn” feature that is personalized to hone the hearing aid settings. The Widex Evoke pro- gram, named “SoundSense Adapt”, learns the user’s prefer- ences in a suitable listening environment. It also allows the user to access and control personalized choices by choos- ing the setting through binary comparisons. It gets smarter by learning from all users by capturing unspecified data preferences and sending them to the Widex Cloud. Sound- Sense also facilitates high-speed processing and automa- tion in the hearing aid [14].
Starkey Livio hearing aids expand their utility to other domains such as tracking daily footsteps, activity level, social listening, and active engagement while interacting to lessen cognitive decline, as well as many other wellness benefits. These advanced features are successful with hear- ing technology that uses integrated sensors and AI. Even though other devices have similar characteristics, research indicates that the ear can receive more accurate informa- tion. A newer version is the Livio Edge AI that provides superior sound quality in challenging listening situations. It has built-in AI technology activated with a simple tap in its Edge mode [15]. An easy way to control it is by using the Thrive Hearing Control app that provides the user with control options [16].
The new Syncro hearing aid released by Oticon includes voice priority processing (VPP) in which AI parallel pro- cessing is employed. Sequential processing may be faster and is able to select the preferred processing option, but it does not compare different outcomes; this can result in a nonoptimal choice of solution due to the unpredict- ability of communication in noisy surroundings. On the other hand, parallel processing means the ability to pro- cess and distinguish different outcomes, providing the best solution. The VPP optimizes speech output and noise reduction by using three mechanisms. Multi-band adap- tive directionality provides a polar pattern for each band of frequency; TriState Noise Management categorizes the noise situations into well-defined listening modes; and voice aligned compression provides compression over an expanded bandwidth based on eight independent chan- nels. These multiple channels optimize the signal pro- vided to the user. In essence, VPP aims to provide the best voice-to-noise ratio using decision-making from the AI parallel processing system [17].
Other AI algorithms used in hearing technology are deep neural networks (DNNs). Deep learning is a sub-field of machine learning that uses algorithms inspired by the brain’s biological structure and functioning to provide machines with intelligence. DNNs became popular in 2019 and their utility in hearing devices began soon after. A recent paper on DNN in hearing aids was by Park et al. [6a] where they found that the DNN could cancel noise by classifying the incoming noise based on its type, thereby providing better speech quality compared to normal hearing aid algorithms. Another article by Lee et al. [7] compared two AI technology algorithms in hearing aids, one a DNN and another a convo- lution neural network (CNN). Their findings were that the CNN outperformed the DNN in terms of its speech enhance- ment strategy [7]. There have been other works document- ing the utility of DNN algorithms in separating speech and noise and allowing speech to be emphasised [18].
Machine learning has also been used in cochlear implants. Some of the machine learning has been used in enhanc- ing speech perception in noise, modelling auditory phys- iology, ambient noise and music processing, automated evoked potential measurement (Auto NRT, Auto ART), signal artifact filtering, post operative performance, surgi- cal anatomy location prediction, electrode placement, and robotic surgery. Most of the algorithms used for these func- tions are deep neural networks, artificial neural networks, support vector machines, decision tree classifiers, linear regression, and Gaussian mixture models. The implant undergoes a training mode in all these situations; after these algorithms are fitted performance becomes more stronger [19-22].
AI has been refined to provide quick and efficient results in cochlear implants [19]. Finding the best setting for the cochlear implant’s speech processor is a challenge, the goal being to provide intelligible speech and good sound infor- mation without relying on visual cues. AI fits the variabil- ity with adaptive intelligence. The Med-El cochlear implant with Rondo 3 speech processor also has adaptive intelli- gence technology. The new version with Automatic Sound Management 3.0 automatically adjusts to the sound in the environment to provide better hearing [23,24].
Other sound processors such as the Cochlear Kanso 2 and Nucleus N7 have novel algorithms such as SmartSound iQ with SCAN technology. It is designed to provide natural hearing by capturing sound and adjusting it to the envi- ronment (speech, noise, speech in noise, wind, quiet, music) [25-27]. To compensate for the cocktail party effect, ForwardFocus helps to focus sound and reduce background noise, especially in face-to-face interactions.
Smart technology can remember settings and programs used in different environments. With the preferred choices, it can scan the environment and provide the best hearing sensation accurately. A variety of sound combinations such as enjoying music, lyrics, speech in quiet, listening effort- lessly and comfortably to the conversation in the presence of noise have paved the way for Advanced Bionics Auto- Sound OS with HiResolution Sound that adapts intelli- gently and automatically for every listening situation [28] .
AI is ubiquitous in mobile phones, computers, and tablets. With the wireless connecting link between these appli- ances and hearing devices, Smartphone compatibility has given rise to innumerable benefits [29]. Using the AI of such devices can bestow quality listening even if it’s tele- vision or music listening on smartphones. It helps to tailor the auditory listening with personal preferences depend- ing on the use [6].
A notable state-of-the-art technology must be Elon Musk’s Neuralink, a neuroprosthesis with a ‘Neural Lace’ technol- ogy. It is a brain-computer interface that allows people to control wired devices for motor activities. This has expanded for auditory capabilities as the chip allows the user to ‘hear’ similar to an auditory cortical implant. Other benefits such as streaming music effortlessly into the brain are evolving faster and will soon be put into practice [30-32].
Conclusion
This short review has given an insight into the utility of the various machine learning techniques used in hearing devices. There is a need to incorporate machine learning algorithms into hearing devices as it improves speech per- ception in noise, enables automation, accelerates the time it takes to process information, eases the job of audiologists, and aids the comfort of individuals using these devices. The utility also extends to surgery and intra-op monitor- ing, where it reduces errors. The literature has also shown an improvement in performance with the incorporation of these algorithms. Currently it may not be feasible for every individual to afford hearing devices with advanced features, but in the near future these devices will become affordable and improve quality of life.
References
1. Copeland J. Artificial Intelligence: A philosophical introduction. John Wiley & Sons, 2015.
2. Copeland BJ, Proudfoot D. Artificial intelligence: history, foun- dations, and philosophical issues. In: Philosophy of Psychology and Cognitive Science, North-Holland, 2007, pp.429–82.
3. Saleh Z. Artificial Intelligence: Definition, ethics and standards. The British University in Egypt, Cairo, 2019.
4. Schum DJ. Artificial intelligence: the new advanced technology in hearing aids. Audiology Online, 2004:14-06.
5. Aliabadi M, Farhadian M, Darvishi E. Prediction of hearing loss among the noise-exposed workers in a steel factory using artificial intelligence approach. Int Arch Occupat Env Health, 2015 Aug 1;
88(6): 779–87.
6. Wolfgang K. Artificial intelligence and machine learning: push- ing new boundaries in hearing technology. Hear J, 2019 Mar 1; 72(3): 26–7.
6a. Park G, Cho W, Kim K-S, Lee, S. Speech enhancement for hear- ing aids with deep learning on environmental noises. Appl Sci- ences, 2020; 10: 6077.
7. Lee YC, Chi TS, Yang CH. A 2.17mW acoustic DSP processor with CNN-FFT accelerators for intelligent hearing aided devic- es. IEEE International Conference on Artificial Intelligence Cir- cuits and Systems (AICAS) 2019 Mar 18, pp. 97–101.
8. Townend O, Nielsen JB, Ramsgaard J. Real-life applications of machine learning in hearing aids. Hear Rev, 2018; 25(4): 34–7.
9. Zhang T, Mustiere F, Micheyl C. Intelligent hearing aids: the next revolution. 38th Annual International Conference of the IEEE En- gineering in Medicine and Biology Society (EMBC), 2016 Aug 16, pp.72–6.
10. How machine learning revolutionizes hearing aids. Signia Hear- ing Aids, 2019. Available from: https://www.signia-hearing.com/ blog/machine-learning-in-hearing-aids/
11. Conde T, Gonçalves ÓF, Pinheiro AP. Stimulus complexity mat- ters when you hear your own voice: attention effects on self-gen- erated voice processing. Int J Psychophysiol, 2018 Nov 1; 133:
66–78.
12. Mesgarani N. Brain-controlled hearing aids for better speech per- ception in noisy settings. Hear J, 2019 Sep 1; 72(9): 10–2.
13. Flynn MC, Lunner T. Clinical verification of a hearing aid with artificial intelligence. Hear J, 2005 Feb 1; 58(2): 34–8.
14. Townend O, Nielsen JB, Balslev D. SoundSense Learn: Listening intention and machine learning. Hear Rev, 2018; 25(6): 28–31.
15. Starkey Hearing. Livio Edge AI. Available from: https://www.starkey. com/hearing-aids/livio-edge-artificial-intelligence-hearing-aids
16. Starkey Hearing. Welcome to the leading edge of hearing technology. Available from: https://www.starkey.com/blog/ articles/2020/03/introducing-livio-edge-ai
17. Flynn, MC. Maximizing the voice-to-noise ratio (VNR) via voice priority processing. Hear Rev, 2004 Apr 8; 11(4), 54–9.
18. Xu Y, Du J, Dai LR, Lee CH. A regression approach to speech en- hancement based on deep neural networks. IEEE/ACM Transac- tions on Audio, Speech, and Language Processing, 2014 Oct 21; 23(1): 7–19.
19. Crowson MG, Lin V, Chen JM, Chan TC. Machine learning and cochlear implantation: a structured review of opportunities and challenges. Otol Neurotol, 2020 Jan 1; 41(1): e36–45.
20. Van Dijk B, Botros AM, Battmer R-D, et al. Clinical results of Au- toNRT, a completely automatic ECAP recording system for co- chlear implants. Ear Hear 2007; 28: 558–70.
21. Feng G, Ingvalson EM, Grieco-Calub TM, et al. Neural preser- vation underlies speech improvement from auditory deprivation in young cochlear implant recipients. Proc Natl Acad Sci USA, 2018; 115: 1022–31.
22. Tan L, Holland SK, Deshpande AK, Chen Y, Choo DI, Lu LJ.
A semi-supervised support vector machine model for predicting the language outcomes following cochlear implantation based on preimplant brain fMRI imaging. Brain Behav, 2015; 5: 1–25.
23. Kim H, Kang WS, Park HJ, Lee JY, Park JW, Kim Y, Seo JW, Kwak MY, Kang BC, Yang CJ, Duffy BA. Cochlear implantation in postlingually deaf adults is time-sensitive towards positive out- come: prediction using advanced machine learning techniques. Scientific Reports, 2018 Dec 20; 8(1): 1–9.
24. Med-El. RONDO 3 Audio Processor. Available from: https://www.medel.com/hearing-solutions/cochlear-implants/rondo3
25. Ashburn-Reed S. Cochlear unveils Kanso™, a first-of-its-kind hearing technology to treat severe to profound hearing loss. 2016 Sep 16. Available from: https://pronews.cochlearamericas. com/cochlear-unveils-kanso-a-first-of-its-kind-hearing-techno- logy-to-treat-severe-to-profound-hearing-loss/
26. Warren C, Nel E, Kwok B. Nucleus 7 moves forward: a new noise reduction algorithm. J Hear Sci, 2018; 8(2): 91–2.
27. Cochlear. Kanso 2 Sound Processor. Available from: https:// www.cochlear.com/us/en/home/products-and-accessories/ nucleus-system/nucleus-sound-processors/nucleus-kanso-2
28. Hazama M, Sasaki M, Nakahara K, Hojo T, Sakoda T, Kawano A. Efficacy of speech in noise using Naida Q90 CI, benefit of speech perception in noise with the latest noise reduction algo- rithm. J Hear Sci, 2018 Jun 1; 8(2): 174.
29. Warren CD, Nel E, Boyd PJ. Controlled comparative clinical trial of hearing benefit outcomes for users of the Cochlear™ Nucleus® 7 Sound Processor with mobile connectivity. Cochlear Implants
Intl, 2019 May 4; 20(3): 116–26.
30. Musk E. An integrated brain–machine interface platform with thousands of channels. J Medical Internet Research, 2019; 21(10): e16194.
31. Kulshreshth A, Anand A, Lakanpal A. Neuralink: an Elon Musk start-up achieve symbiosis with artificial intelligence. Interna- tional Conference on Computing, Communication, and Intelli- gent Systems (ICCCIS), 2019 Oct 18, pp. 105–9.
32. Umashankar A, Prabhu P. Can Neuralink be effective for bionic hearing? [online blog] Hear J, 2020; 73(12).