Makina Öğrenimi ile ABR Cevaplarından Tinnitus Tahmini Yapmak
- engindani5
- 24 May 2022
- 2 dakikada okunur
Predicting tinnitus from auditory brainstem responses using machine learning
Authors: Jessica J. M. Monaghan1, Zhe Liu2, Lina Yao2, and David McAlpine3
1National Acoustic Laboratories, Sydney, Australia,
2School of Computer Science and Engineering, The University of New South Wales, Sydney, Australia
3Department of Linguistics, Macquarie University, Sydney, Australia
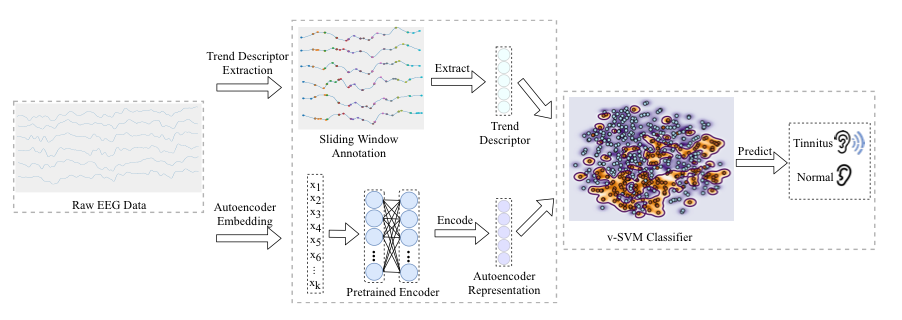
Background: Tinnitus is the perception of sound in the absence of a sound source. Currently, no objective measure for diagnosing tinnitus exists, relying instead on self-report. This renders a definitive diagnosis difficult, limits the efficacy of potential treatments, and reduces the possibility for redress following events, such as unwanted exposure to loud sounds, that might contribute to the onset of a tinnitus percept. Here, we apply machine learning to distinguish—at the individual level—those who report a perception of tinnitus from those who do not, based on their auditory brainstem responses (ABRs).
Methods: The algorithm was trained and tested on a dataset from Schaette and McAlpine (2011), which comprised 15 participants with tinnitus and 18 controls. Participants had matched and normal thresholds—including for frequencies higher than assessed by standard audiometry—and were controlled for age/gender. Classification was challenging because of the small amount of training data available, as well as the high degree of inter-subject variability and low signal-to-noise ratio typical for ABRs. A novel Siamese-autoencoder approach was developed to overcome these difficulties by generating a low-dimensional representation of the data that maximised the differences between tinnitus and control data. Together with a trend-descriptor that provided a robust summary of the temporal features of the signal, the auto-encoder representation was provided as input to a support-vector machine classifier.
Results: Accuracy over 80% was achieved.
Conclusions: This indicates the existence of objective features in neural activity generated by the inner ear and early auditory brain that differ between individuals with and without subjective tinnitus. Our current method achieves relatively high levels of accuracy from ABR data using very limited data. This approach shows great potential to be developed into a diagnostic tool.
Schaette, R., & McAlpine, D. (2011). Tinnitus with a normal audiogram: physiological evidence for hidden hearing loss and computational model. Journal of Neuroscience, 31(38), 13452-13457
Comments