YZ yardımı ile Orta Kulak Patolojilerinin Tanılanması
- engindani5
- 24 May 2022
- 2 dakikada okunur
AI-assisted Diagnosis for Middle Ear Pathologies
Authors: Jaco Verster1, Hermanus Carel Myburgh1,2, Claude Laurent1,3,4, De Wet Swanepoel1,3 1hearX SA (Pty) Ltd, Building 2, Ashlea Gardens Office Park, 180 Garsfontein Road, Ashlea Gardens, Pretoria, 0081, South Africa. Email: jaco@hearxgroup.com 2Department of Electrical, Electronic and Computer Engineering, University of Pretoria, South Africa, C/o Lynnwood Road and Roper Street, Hatfield, Pretoria, 0002, South Africa. Email: herman.myburgh@up.ac.za 3Department of Audiology and Speech-Language Pathology, University of Pretoria, South Africa, C/o Lynnwood Road and Roper Street, Hatfield, Pretoria, 0002, South Africa. Email: dewet.swanepoel@up.ac.za 4Department of Clinical Science, Otorhinolaryngology, Umeå University, Umeå, Sweden. Email: claude.laurent@umu.se Background: Between 65 and 330 million people are affected annually by chronic otitis media (OM) globally. While OM is one of the most common childhood illnesses, it is also the most common reason for doctor visits. If misdiagnosed or left untreated, it may cause adverse side-effects and even result in death. Many third world countries do not have access to ear and hearing specialists while specialist equipment is also in short supply. There is a need for a commercial, automated, assistive diagnosis system which can be used by inexperienced medical professionals to make accurate diagnoses of OM, to provide efficient care and prevent secondary complications. Method: The current artificial intelligence (AI) assisted diagnosis system has been developed over the past five years. This system consists of a custom developed USB digital video otoscope and Android application that connects to a cloud server via the internet. The mobile application allows for real-time image capture and facilitates telemedicine diagnosis. Once an image is captured, it is sent to the server and first classified as a valid or invalid ear using a convolutional neural network (CNN). The image is then diagnosed using an ensemble of deep CNN’s and the result is returned to the smartphone. Currently, captured images are diagnosed into four classes (Normal, Wax obstruction, Perforation and Abnormal) with high accuracy. CNN training is accomplished by using a transfer learning approach based on 1544 images pre-diagnosed by at least two experienced ENTs.

The digital video otoscope with mobile phone and AI-assisted diagnosis software.
Results: The overall weighted average accuracy of the AI-assisted diagnosis system is 97%. The recall/sensitivity of the respective diagnostic classes are as follows: Normal (99.33%), Wax obstruction (96.26%), Perforation (97.10%), Abnormal/Other (93.33%). Users of the system have reported extreme ease-of-use while diagnoses are quick (<5 s) with a stable internet connection.
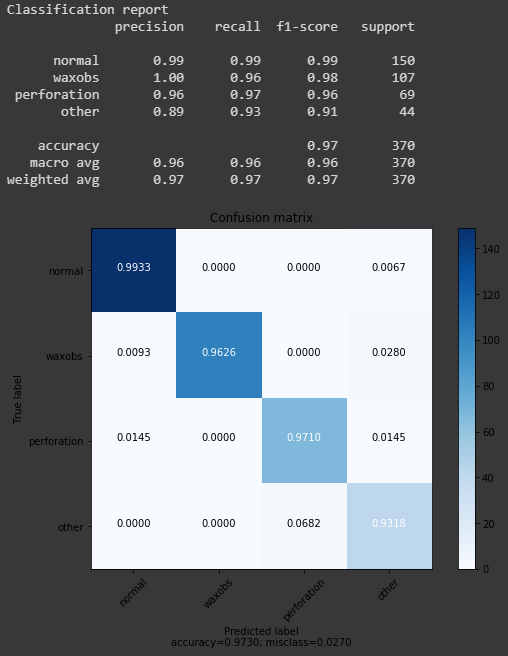
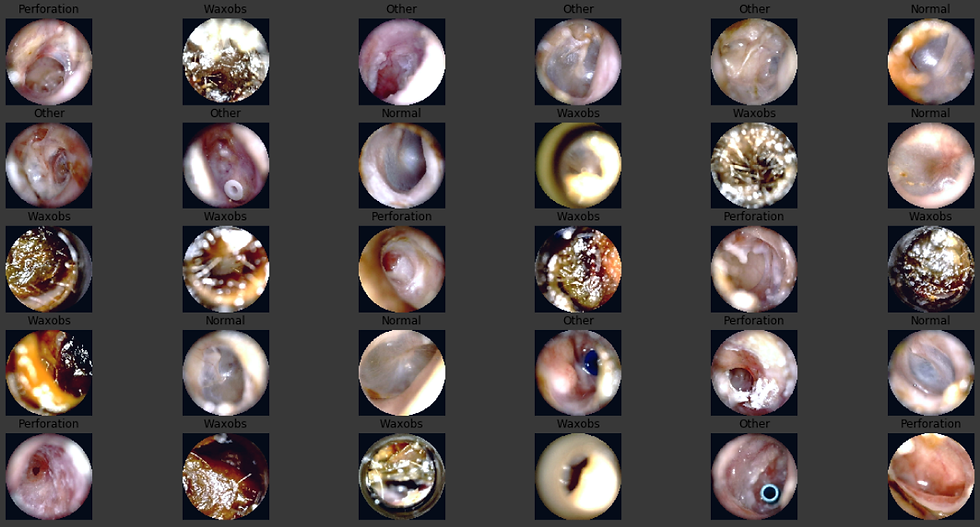
Simulated CNN results (top) and sample diagnosed images (bottom)
Conclusion: An AI-assisted diagnosis system for commercial use was developed and released as a public beta version. The system can be used on any suitable Android smartphone or tablet device with internet access, using the mobile application and custom-developed video otoscope. As more images are being captured, future versions will expand the abnormal category by specifying various pathologies including, acute otitis media, cholesteatoma, myringosclerosis and otitis media with effusion.
Commentaires